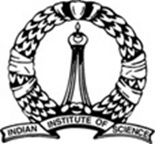 |
Modeling urban dynamics along two major industrial corridors in India |
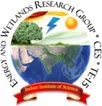 |
Materials and Methods
3.1 Study Area
Assessment of land use dynamics and prediction of likely land uses in the future based on agent-based modeling has
been carried out in the major industrial corridors connect-ing two metropolitan cities of India—Mumbai–Pune Industrial (MPI) corridor known as Yashwantrao Chavan Mumbai–Pune expressway and Chennai–Bangalore–Man-galore Industrial (CBMI) corridor.
MPI corridor (Fig. 1a) is India’s first six-lane high-speed toll express highway extending over a distance of Ninety-three kilometres connecting Mumbai, also known as ‘‘Business Capital of India’’ and Pune ‘‘Industrial and Educational Hub’’ and also known as ‘‘Manchester of India’’.
CBMI corridor extends along the National Highway (NH 48 and NH4) covering a distance of 630 km (Fig. 1b). The Government of India extended Chennai–Bangalore industrial corridor to Mangalore in order to connect east and west ports. The industrial corridor connects the major Tier I cities such as Chennai and Bangalore and Tier II city Mangalore.
The buffer of 10 km on either side was considered to understand the effects of the industrial corridor in its immediate neighbourhood. The study region of MPI is about 3022 sq km covering districts of Mumbai, Raigarh, Thane and Pune. The CBMI study region is about 13,572.37 km2 and the industrial corridor passes through 15 districts including Chennai, Chittoor, Dharmapuri, Kancheepuram, Thiruvallur, Vellore, Thiruvannamalai,
Bangalore, Bangalore Rural, Hassan, Mandya, Tumkur, Dakshina Kannada and Kasargod.
3.2 Data and Method
Figure 2 outlines the method which includes three-step framework. The first step includes data acquisition, pre-processing and land use land cover change analysis (LULCC). Data acquisition involves the collection of Pri-mary data and Secondary data. Primary data includes remote sensing data for the period between 1997 and 2015 [34]. Table 1 lists the data obtained from various sources. Secondary data were used to support primary data for geometric corrections and land use classification, which includes historical land use maps, the Survey of India Topographic maps [35], information from virtual earth database viz., Google Earth [36] and Bhuvan [37]. Ground control points were collected with hand held pre-calibrated GPS (Global Positioning System).
The remote sensing data was preprocessed for geometric/positional errors and geometric correction was carried out using GCPs for inaccurate datasets. Radiometric cor-rections were carried out to enhance spectral properties of the chosen scene. All data were resampled to common spatial resolution i.e., 30 9 30 m to maintain uniformity across the temporal data. The study region (25 km length corridor with 10 km buffer on either side of the corridor) was delineated/cropped from the respective scene.
The land cover analysis was performed using Normalised difference vegetation index (NDVI) to understand the spatial extent of region under vegetation and non-vegetation. NDVI values ranges between - 1 (non-vege-tation) and ? 1 (vegetation). Remote sensing data was classified considering supervised classifier based on Gaussian maximum likelihood algorithm. This included the creation of false color composite (FCC), which aided in identifying heterogeneous features, training polygons were chosen from these representative heterogeneous features. These training polygons with geo co-ordinates were loaded in pre-calibrated (error \ 1 m) GPS and the corresponding attribute data for the training polygons were collected from the field. These data were then used to classify remote sensing data into four classes (Table 2) using a supervised classifier based on the Gaussian maximum likelihood algorithm and this technique was found superior in terms of classification accuracies [38]. Validation of remote sensing data classification is done through accuracy assessment using overall accuracy and kappa statistics [39].
The study region (respective industrial corridor with 10 km buffer on either side) was segmented into 25 km zones to understand the pattern and process of urbanisation in the neighbourhood. Initially, the region was segmented at every 5 km interval, 10 km, 25 km and 50 km and spatial indices were computed. It was found that 25 km was optimal to understand the spatial patterns of landscape dynamics using metrics.
Then, modeling of likely growth was simulated using CA-Markov integrated with Fuzzy ABM framework. Figure 2 explains the entire process of analysis and visual-ization based on fuzzy logic with AHP, MCE and CA.
Fuzzy Logic helped in understanding the behaviour of agents, the Analytical Hierarchical Process (AHP) aided in assessing the role of each agent. This was combined with the already proven technique of Multi-Criteria Evaluation (MCE), Markov chains and Cellular Automata (CA) to model and visualize the urban growth. Agents that facili-tate the urban growth process are roads, industries, edu-cational institutions, bus stands, railway stations, metro, population, etc. Ecosystems (such as water bodies) that are to be conserved for the sustenance of natural resources are considered as constraints. Validation of the simulated growth was performed with the actual growth (2014/2015) through kappa indices, as a measure of agreement. Once these data and agents are trained and validated, data is used to model and simulate for future trends (10 years) with definite time steps.
Anthropogenic and topographic parameters were used to evaluate the probability of each pixel converting to urban in the study area using the fuzzy AHP method. AHP was computed as per Saaty 2011 [40]. The weight of the effective factors was estimated based on fuzzy AHP with the creation of 25 different judgment scenarios. Each sce-nario had six pairwise matrices (agents) including the development of sub-matrices (sub agents). Variables were developed similar to fuzzy triangular numbers and mean was considered based on fuzzy extent analysis [41] to get the weights of each agent that included fuzzy comparison and then fuzzy arithmetic mean, development of degree of possibility and developing priority vector. The consistency ratio (CR) was calculated to evaluate the accuracy of the derived weights.
Classified land uses were verified by comparing the predicted land use with the actual land uses. Suppose, 3 years y0, y1 and y2 land uses are computed through supervised classifier, first, two land uses of y0, and y1 were used to train, calibrate and predict the land use for the year y3. These predicted values were compared with the actual land use as per the classified information of y3. The accuracy of prediction is assessed using Kappa statistics as a measure of agreement between predicted y3 and actual y3 (classified).
|
|
Citation :T.V. Ramachandra, Jeffery M. Sellers, H. A. Bharath, S. Vinay, 2018. Modelling urban dynamics along two major industrial corridors in India. Spatial Information ResearchISSN 2366-3286Spat. Inf. Res.DOI 10.1007/s41324-018-0217-8
|